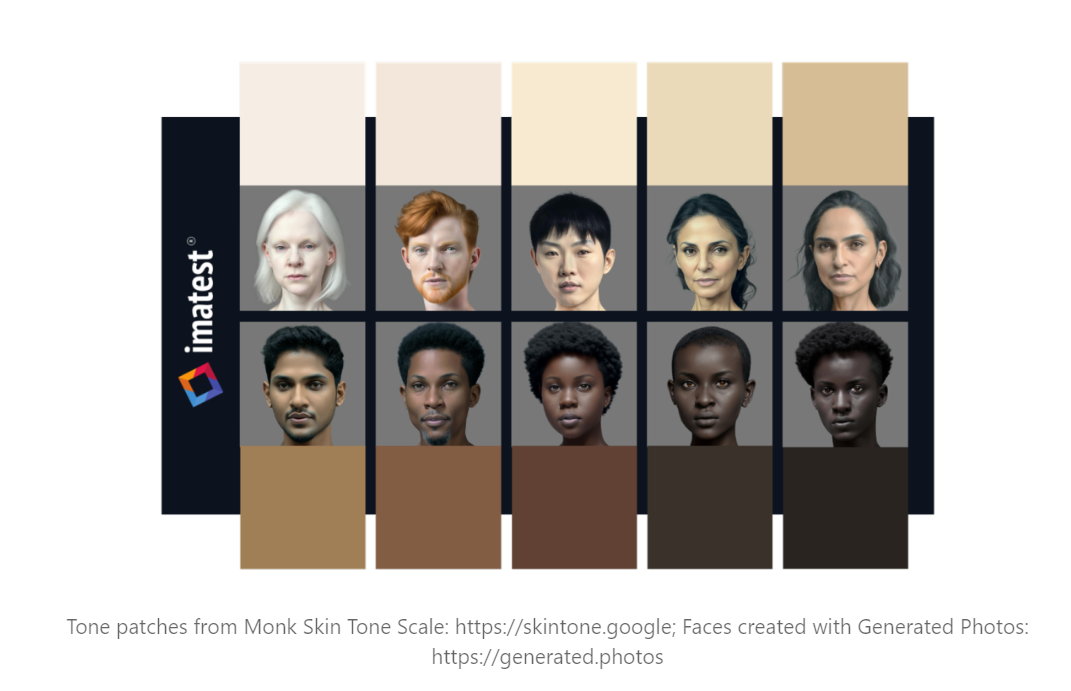
by Meg Borek
We should be designing more equitable cameras.
I recently tested a variety of webcam and smartphone devices as part of a project that I presented at this year’s Electronic Imaging Symposium, hosted by the Society for Imaging Science and Technology (IS&T). I was disappointed by the results, which showed consistently poor performances across scenes containing darker skin tones. But I realized that this is a problem that others have known of and experience every day—something I have long taken for granted. And that’s not okay.
There are many compelling articles that describe the historical background of racial bias in photography. One written by Professor Sarah Lewis at Harvard and another published by Google regarding their Real Tone technology are a few good reads to start with. The moral of these stories? We can do better.
The saying “the camera cannot lie” is almost as old as photography itself. But it’s never actually been true. ~ Google
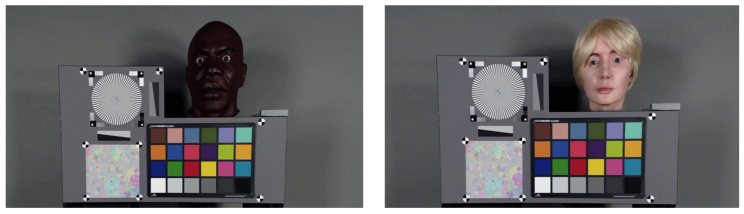
Richard (left) and Alexis (right) mannequins captured with same $50 web camera under identical lighting conditions.
Meet two of our test mannequins, Richard (left) and Alexis (right) at Imatest. Both of the above frames are captured with the same $50 USD webcam under the same illumination conditions, using out-of-the-box default settings. If you’re curious about the chart configuration, check out the Valued Camera Experience (VCX) WebCam Specification. Data capture and analyses in this project were based on procedures defined by VCX, but we pay particular attention to the ColorChecker and the faces for this study. This particular chart is a prototype designed at Imatest.
Right off the bat, we see that the darker skin tone is underexposed in comparison to a lighter skin tone under the same lighting conditions. If we calculate the average Delta E 2000 (CIEDE2000) error for the ColorChecker in each scene, compared in the figure below, we’ll find that both have nearly identical errors. But this doesn’t consider the appearance of each face. None of this is particularly surprising.
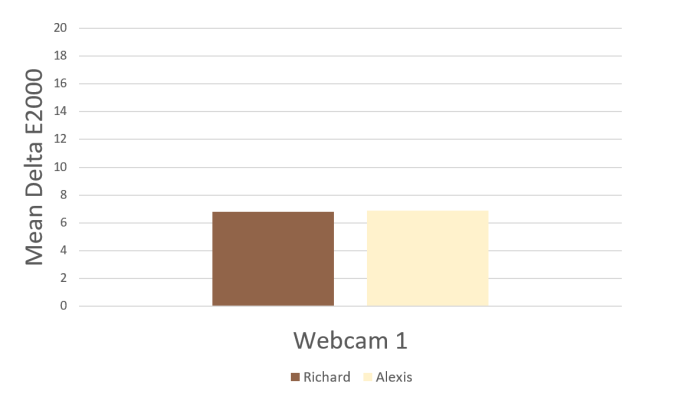
Average Delta E error across all patches of the ColorChecker chart in Richard vs Alexis scenes
But let’s make the automatic exposure algorithm “smarter” in the next example. These frames are captured by a $300 USD webcam that uses face-detection to steer its exposure settings.
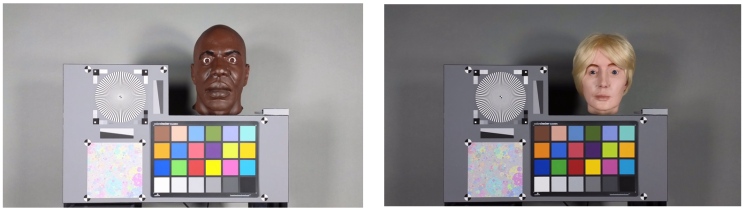
Richard (left) and Alexis (right) mannequins captured with a face-detection $300 USD web camera under identical lighting conditions.
Big difference—we can actually see details in Richard’s face. But there’s a big trade-off.
The camera has automatically adjusted the settings to better expose whatever face it finds in the scene. That’s an improvement, but it introduces a new problem for darker skin tones: now everything else is overexposed.
We can analyze the ColorChecker chart from the two scenes in Imatest to produce the simulated charts below. Each tile shows a split view of the target/measured colors for each patch. We see oversaturation in several of the patches from the Richard scene (highlighted in red), but in none of the patches in the Alexis scene.
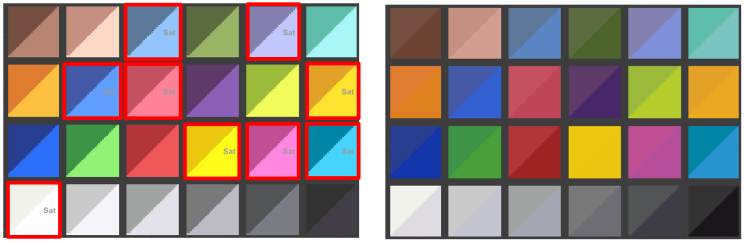
Simulated ColorChecker charts comparing reference/measured patch colors for the Richard (left) and Alexis (right) scenes captured by the face-detection webcam.
Consequently, the average Delta E error of the ColorChecker in the Richard scene increases dramatically.
I tend to hear the argument that this is a good thing—prioritizing proper exposure of a face in the scene. But that seems incredibly unfair when most cameras are able to properly expose all areas of scenes containing lighter skin tones. Image processing algorithms are not a “one-size-fits-all” situation.
But okay. It’s a webcam. Do people even turn those things on during virtual meetings anymore? Who’s even looking that closely?
Though something we do look closely at is our smartphones, and the videos and photos that they produce. So let’s make the automatic algorithms even smarter.
Modern smartphone exposure and color reproduction algorithms are stepping away from the use of global adjustments, i.e., increasing the exposure to prioritize the face doesn’t increase the exposure over the entire image. Here’s an example captured with my own phone:
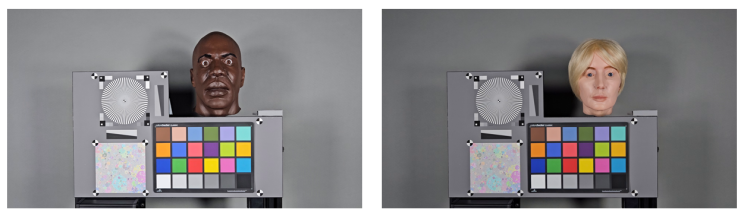
Richard (left) and Alexis (right) mannequins captured with a modern smartphone in identical lighting.
The visual difference is more subtle, but the underlying problem still exists, especially when we look at the numbers.
What else does this mean? Uniform patches on the ColorChecker probably aren’t the best way to gauge skin tone reproduction, especially for processing pipelines that utilize face-detection.
But so far we’ve only looked at two skin tones. Let’s test more.
Using AI to Generate Diverse Human Faces
The Monk Skin Tone (MST) Scale was developed by Dr. Ellis Monk at Harvard and is comprised of 10 different tones. The scale is currently being used by Google Research and is designed to represent a broader range of geographic communities than the commonly used Fitzpatrick scale, which is skewed towards lighter skin tones due to its dermatological background.
I didn’t have access to a diverse group of real people for this study, but we do have the impressive powers of AI now. I worked with Generated Photos to create 10 AI-generated faces with skin tones based on the Monk scale. The company’s Human Generator tool is pretty cool. It offers:
- 16 base skin tone options
- 120+ ethnicities
- Custom AI prompt input
- Dozens of pose, clothing, and background options
Initial parameters were chosen to most closely match the target skin tones, followed by manual color adjustments in Adobe Photoshop before being printed with color-accurate printing methods at Imatest. Spectral reflectance measurements of each simulated skin tone are compared to those of actual human skin (collected by NIST) below, if you’re curious how they compare.
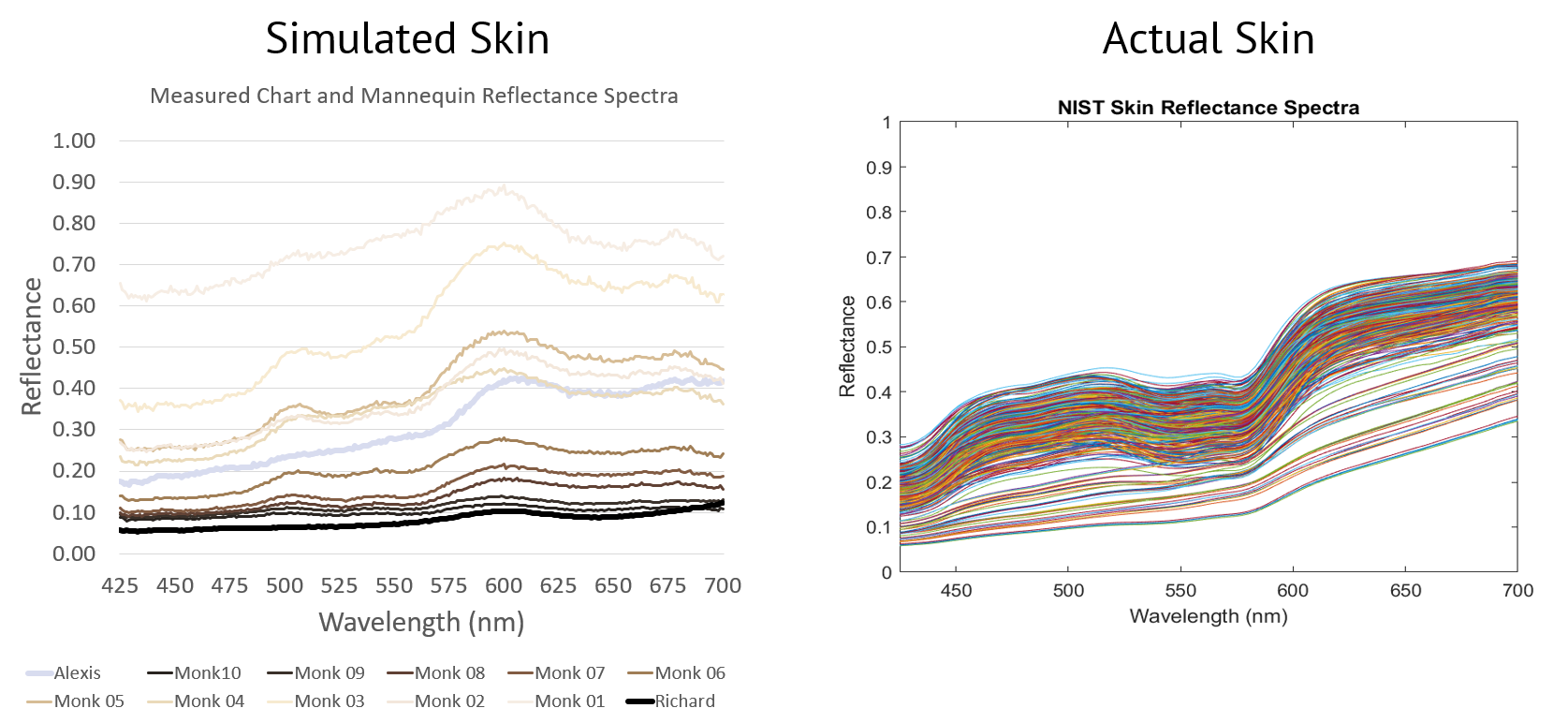
Spectral reflectance measurements of mannequins and simulated face charts (left) compared to reflectance spectra of actual skin, collected by NIST (right)
Then it was time to capture a lot of data.
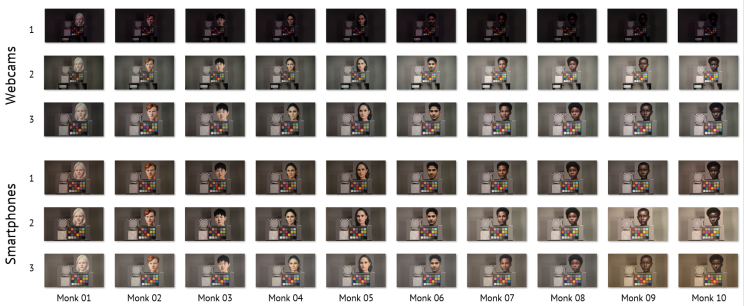
Monk Skin Tone scenes captured by 6 test devices at 20 lux, Illuminant A
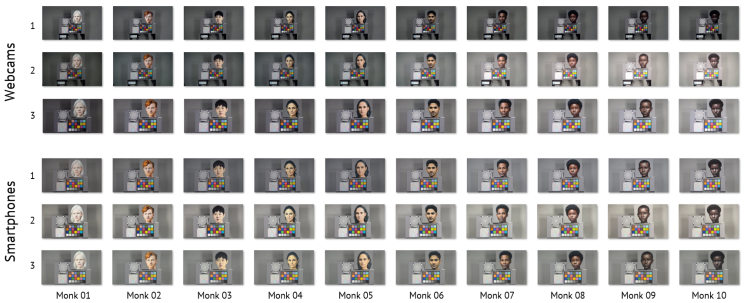
Monk Skin Tone scenes captured by 6 test devices at 250 lux, 6200K CCT
Subsets of the captured data for two illumination conditions (out of 15+ tested) are shown above. Notice the trend in exposure in the scenes from left (lightest Monk skin tone) to right (darkest Monk skin tone). All but Webcam 1 utilize some form of face-detection, and we tend to see an increase in overall scene brightness as the skin tone gets darker. We also notice some white balance errors, which are discussed more in depth in my conference proceedings (coming soon!) and in the VCX specification.
The Results
Again, we calculate the average Delta E error of the ColorChecker in each scene. We also compute the relative error of the average lightness, or L* value, of the face in each scene. With these two metrics, we can, in a very simplified way, compare how good the chart (or surrounding scene) looks versus how good the face looks.
If we plot these two metrics against one another for each device and each scene, we get a very telling scatter plot:
What do we see? Errors tend to be noticeably larger for scenes containing darker skin tones. It also shows how the handling of darker skin tones varies more drastically within and between devices. The errors in scenes containing darker skin tones are far more dispersed than those for scenes containing lighter skin tones, which fall within a much tighter range. So not only are scenes containing darker skin tones being handled more poorly, they are also handled inconsistently.
But some camera systems are starting to get it right.
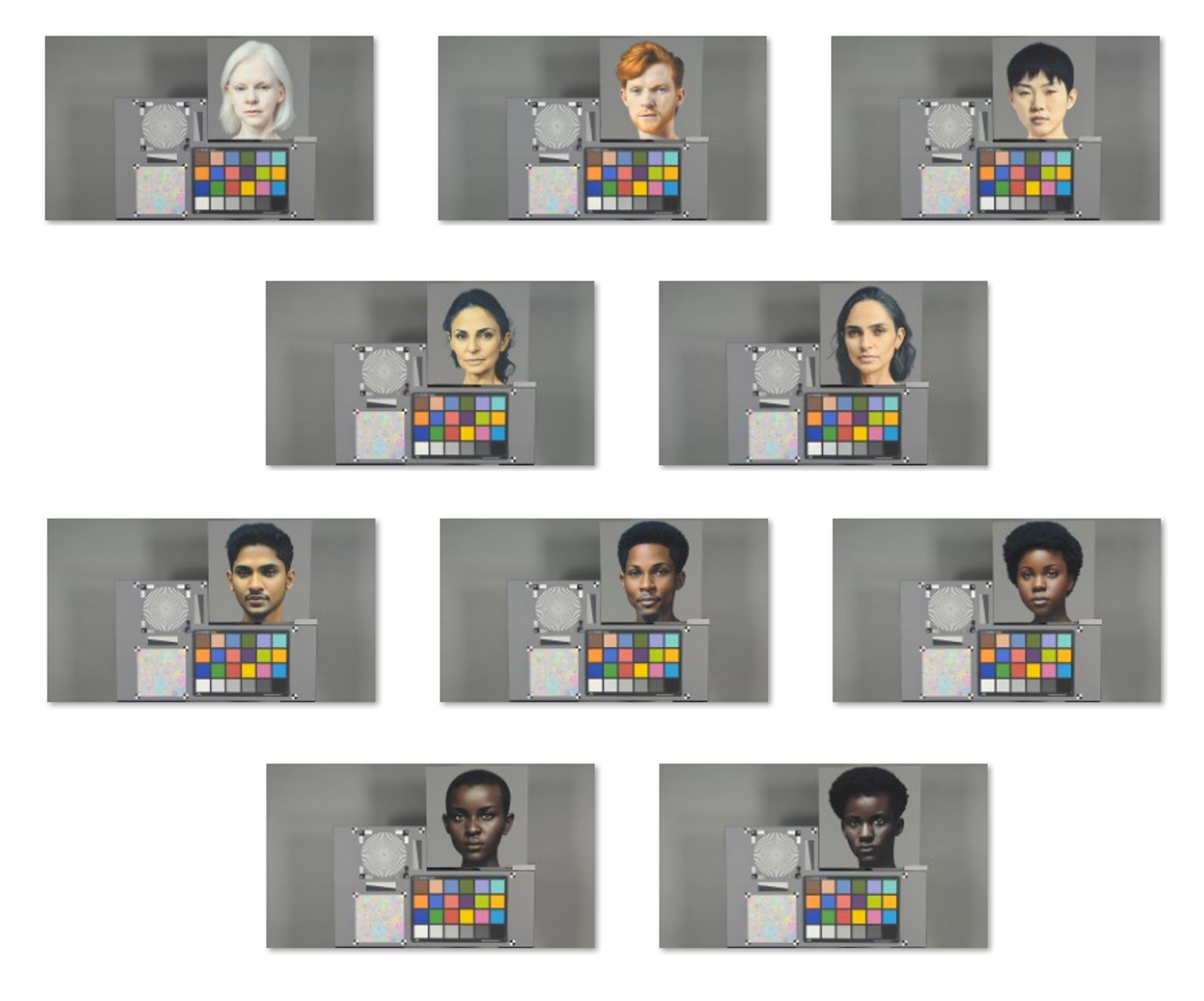
Monk Skin Tone charts captured with the Google Pixel 6 Pro under identical illumination conditions
The scenes above are each captured under identical illumination conditions using default settings on the Google Pixel 6 Pro. The Pixel 6 is Google’s first model that incorporates their Real Tone technology, which is an incredible effort to make their photography products perform more equitably across a wide range of skin tones.
A summary of a subset of the results from six devices tested across skin tones and lighting conditions is shown below.
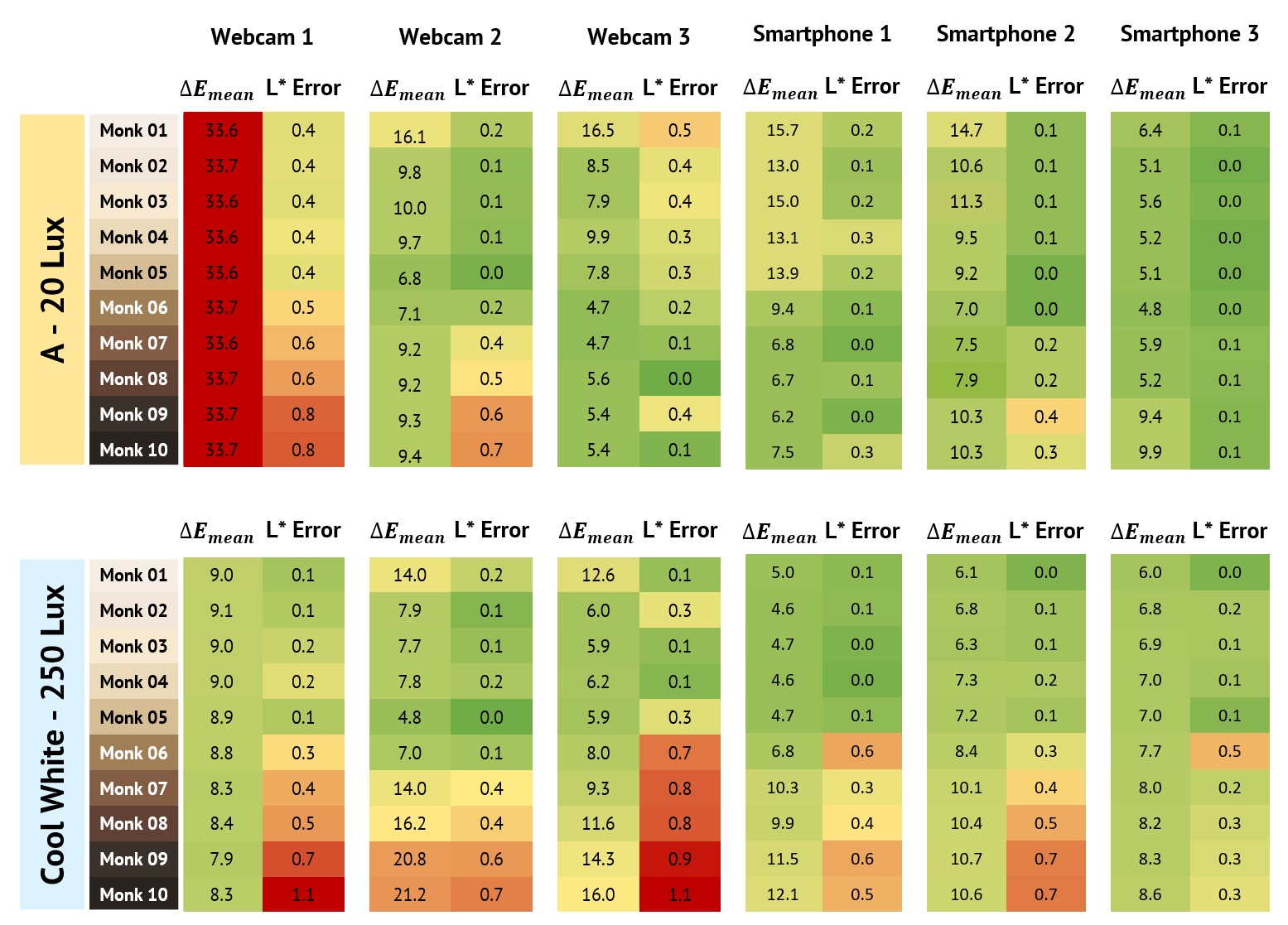
Results summary for Delta E and L* error measured for six devices tested across two lighting conditions and ten skin tones based on the Monk Scale
Despite being more than two years old, the Google Pixel 6 Pro (Smartphone 3) outperforms not only the web cameras, but also both Smartphones 1 and 2, which are recent 2023 models created by two other popular smartphone manufacturers.
What this means for the industry
This article only scratches the surface of a complex issue. It doesn’t even begin to look at scenes containing multiple skin tones or other important metrics such as white balance. But I hope it can at the very least convince you that this is a problem, and that we should be making the effort to design more equitable camera systems.
These efforts need to span multiple areas of the industry—not just in the development of camera systems themselves, but also in the development of the standards that test them. We have efforts like VCX and emerging efforts in ISO to expand the range of skin tones that are tested in standards, but there is still a lot to be done. Proper representation of skin tone diversity in camera and image technologies should not just be appreciated, it should be expected.
This is a summary of the EI2024 Paper “Evaluating Camera Performance in Face-Present Scenes with Diverse Skin Tones”